Artificial intelligence is revolutionizing the way we create content, but this advancement comes with its own set of challenges. A notable issue is the problem of AI 'hallucinations,' where the AI generates statements that are factually incorrect yet presented with undue confidence.
Additionally, AI might not always adhere to the brand guidelines, leading to inconsistencies in voice and style. As the stakes are incredibly high in branded content, ensuring accuracy and compliance is paramount.
Hallucinations present a significant risk for companies that rely on AI for content generation. These are not merely minor errors but can be substantial factual inaccuracies. In sectors like healthcare or finance, hallucinations could have more severe consequences such as disseminating false medical advice or financial guidelines.
Therefore, grounding AI with accurate data is not just beneficial but essential to avoid such liabilities.
Challenges of Using Pre-Trained Language Models
Pre-trained language models come with an inherent set of limitations. These models are trained on large datasets but possess a fixed 'memory,' meaning they can only recall information available up till their cut-off date. This static knowledge base can be restrictive, particularly in industries that rapidly evolve.
Attempts to mitigate these flaws include methods like Reinforcement Learning from Human Feedback (RLHF) and fine-tuning. Despite these efforts, the models can still generate inaccuracies, presenting an ongoing challenge for brands striving to maintain credibility.
AI hallucinations can have specific and wide-ranging detrimental impacts.
One common example involves geographical errors, where an AI might claim a certain landmark is in the wrong location, confusing its audience. Another prevalent issue is in historical contexts; an AI might confidently assert that a particular event occurred in a year different from the actual one, or make up something that didn't happen at all.

Source: Flying Bisons
For brands, such errors not only tarnish credibility but could potentially lead to legal consequences. Hence, mitigating these inaccuracies is crucial for maintaining the integrity of AI-generated content.
How RAG Makes AI Ready for Marketers
Retrieval Augmented Generation (RAG) offers a formidable solution to the shortcomings of conventional AI models. Unlike static pre-trained models, RAG incorporates a dynamic data retrieval system that pulls in real-time information from an extensive array of sources.
At its core, RAG enhances AI by bridging the gap between static language models and dynamic data sources. By incorporating external data, RAG expands the AI's knowledge beyond its initial training, enabling it to adapt to the ever-changing world of marketing.
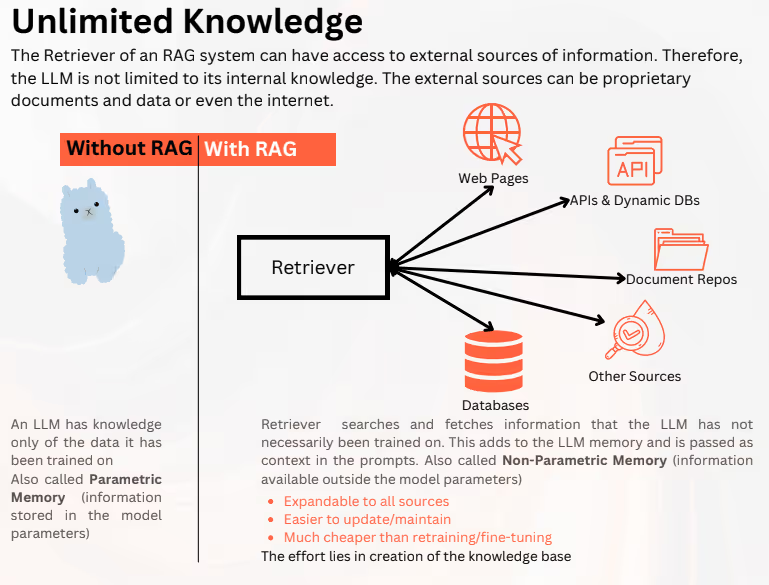
Source: Abhinav Kimothi
The process begins with an AI model generating a query, which is then passed to a retriever system. This system searches external databases to gather relevant information. The retrieved data is then reintroduced into the language model, which generates a final, context-aware output.
There are several methods of implementing RAG, each with its unique advantages.
The versatility of Retrieval Augmented Generation allows it to be applied across a wide range of use cases, each presenting distinct needs and obstacles. Let's explore the different approaches to creating RAG frameworks.
- Naive RAG: This simplest version of technology simply retrieves information and inputs it into language models to improve the richness of the output. While it works well, it might miss the subtle understanding needed for more intricate situations.
- Advanced RAG: This refined approach enhances the basic concept by integrating sophisticated techniques before and after retrieval, offering richer context to the language models. This is especially beneficial in situations that demand a deeper grasp of context.
One notable approach in advanced RAG is the combination of knowledge graphs and RAG. Knowledge graphs provide a structured format for storing and accessing complex data relationships, enhancing the accuracy and depth of the generated content. This synergy results in a robust system capable of delivering precise and contextually rich outputs, tailored to specific requirements.
Yarnit employs this method to achieve unparalleled content accuracy. By integrating a comprehensive knowledge hub with RAG, Yarnit ensures that the AI's output aligns perfectly with brand guidelines, eliminating the risk of inconsistencies or factual errors.
This seamless integration of search and language models ensures that output remains current and factually accurate. RAG not only enriches the content with up-to-date data but also provides contextually relevant answers. Furthermore, knowledge graphs amplify the effectiveness of RAG by organizing data in a structured manner. This combination minimizes the risk of hallucination, ensuring that the AI's output is both accurate and reliable.
Grounding AI : Ensuring Accuracy & Reliability
Grounding AI with data brings a host of advantages, such as:
- Ensuring Accuracy and Reliability: Immediate access to updated information minimizes the risk of inaccuracies, bolstering the credibility of AI-generated content.
- Maintaining Relevance in a Fast-Changing World: RAG empowers AI to remain agile by incorporating the latest data, ensuring that the content stays current and relevant
- Adhering to Brand Guidelines: Grounding AI in data enables it to consistently follow brand guidelines, preserving the brand's voice and expertise.
- Mitigating Risks: With RAG, the risk of disseminating erroneous information is significantly reduced, ensuring the integrity of the content.
- Enhancing User Trust: Accurate, up-to-date content fosters greater trust among users, which is invaluable for brand reputation.
Speaking on the importance of data for marketing usecases, Kalyani Kasara, Lead Global Digital Content & Strategy at Tata Elxsi, said "Marketing has always been a creative, right -brain thinking profession, and I think today it has transformed to being more strategic function. AI is further enhancing that with data being the key."
RAG-Powered AI For Brand Compliance : Marketer's Boon
For marketers, RAG offers a wealth of benefits. It empowers them to create content that is not only engaging but also factually accurate and up-to-date. Imagine being able to generate product descriptions, blog posts, or social media updates that seamlessly incorporate the latest information about your brand, industry trends, or customer feedback. With RAG, marketers can save time and resources while delivering high-quality content that resonates with their target audience.
For instance, a fashion retailer could feed the AI with data about its latest collections, seasonal trends, and customer preferences. The AI would then generate product descriptions, style guides, or promotional content that accurately reflects the brand's identity and resonates with its customer base.
RAG's potential extends far beyond content creation. It can also amplify human effort in areas such as customer service, market research, and data analysis. For example, RAG-powered chatbots can provide instant, accurate responses to customer inquiries by retrieving relevant information from a knowledge base. Similarly, RAG can assist marketers in analyzing vast amounts of data, identifying patterns, and generating insights that inform strategic decision-making.
By grounding AI with real-time data, RAG ensures that the content remains accurate, relevant, and brand-compliant, fostering trust among audiences and strengthening brand reputation. Yarnit employs RAG technology to deliver content that is not only accurate but also aligns impeccably with brand guidelines. By incorporating a comprehensive knowledge hub, Yarnit enriches the AI's memory with detailed, company-specific information.
By inputting brand-specific information into the knowledge hub, marketers can ensure that the AI generates content that adheres to their unique voice, style, and messaging.This eliminates the risks of hallucinations, giving brands peace of mind that their content remains consistent and reliable. Yarnit's application of RAG has also set a new industry standard for brand-compliant, quality content creation.
Yarnit's implementation of RAG is only the beginning. As technology continues to evolve, the potential applications for RAG are manifold. Future iterations of RAG could integrate even more intricate knowledge graphs, allowing for hyper-personalized content that adapts in real-time to new information and user interactions.
Conclusion
Grounding AI with real-time data is essential to ensure the generation of accurate, relevant, and brand-compliant content. Solutions like Retrieval Augmented Generation (RAG) and knowledge graphs offer revolutionary advancements that address the inherent limitations of pre-trained language models, minimizing the risk of errors and maintaining brand integrity.
Yarnit's integration of these technologies exemplifies the future of reliable AI-generated content. Ready to transform your content creation process? Dive into the cutting-edge capabilities of RAG-powered AI with Yarnit and elevate your brand's storytelling to unparalleled heights.